What is Retrieval Augmented Generation and Why You Should Care
One of the main challenges in the widespread implementation of AI is the issue of trust. While artificial intelligence has made significant advancements, it still faces limitations and obstacles that hinder its reliability and accuracy in knowledge-based work.
ChatGPT, for instance, is known for its impressive abilities and convincing responses. However, it has gained notoriety for generating false or misleading information, which are referred to as "hallucinations." These hallucinations pose a serious problem in tasks that require precision and accuracy because relying on AI output without verification can lead to costly mistakes and misinformation.
The Challenge for Knowledge-Based Work
Many jobs that rely on knowledge involve analyzing internal and external information, processing it, and generating new insights based on that knowledge. In such roles, it is crucial to have reliable and trustworthy AI tools, especially during critical decision-making processes.
With tools like ChatGPT, it becomes challenging to determine the accuracy and reliability of the generated response without external verification. This uncertainty hampers productivity and acts as a barrier to fully harnessing the potential of AI in knowledge-based work.
Do language models dream of electric sheep?
To comprehend why AI systems like ChatGPT experience hallucinations, it is necessary to delve into the functioning of large language models (LLMs) employed by companies like OpenAI , Google , and Anthropic. LLMs are trained on vast sets of data to learn patterns and predict the next word in a sentence. While this approach enables LLMs to generate coherent and contextually relevant responses, it also exposes them to potential biases and stereotypes present in the training data.
Due to these biases, AI systems may produce responses that reinforce them, even if they are statistically correct. For example, when I asked Google's Bard about my educational background, it confidently answered that I attended Berkeley and Stanford, as individuals with profiles similar to mine tend to go to these universities. However, I actually went to a different university, Erasmus, and did not attend Berkeley or Stanford.
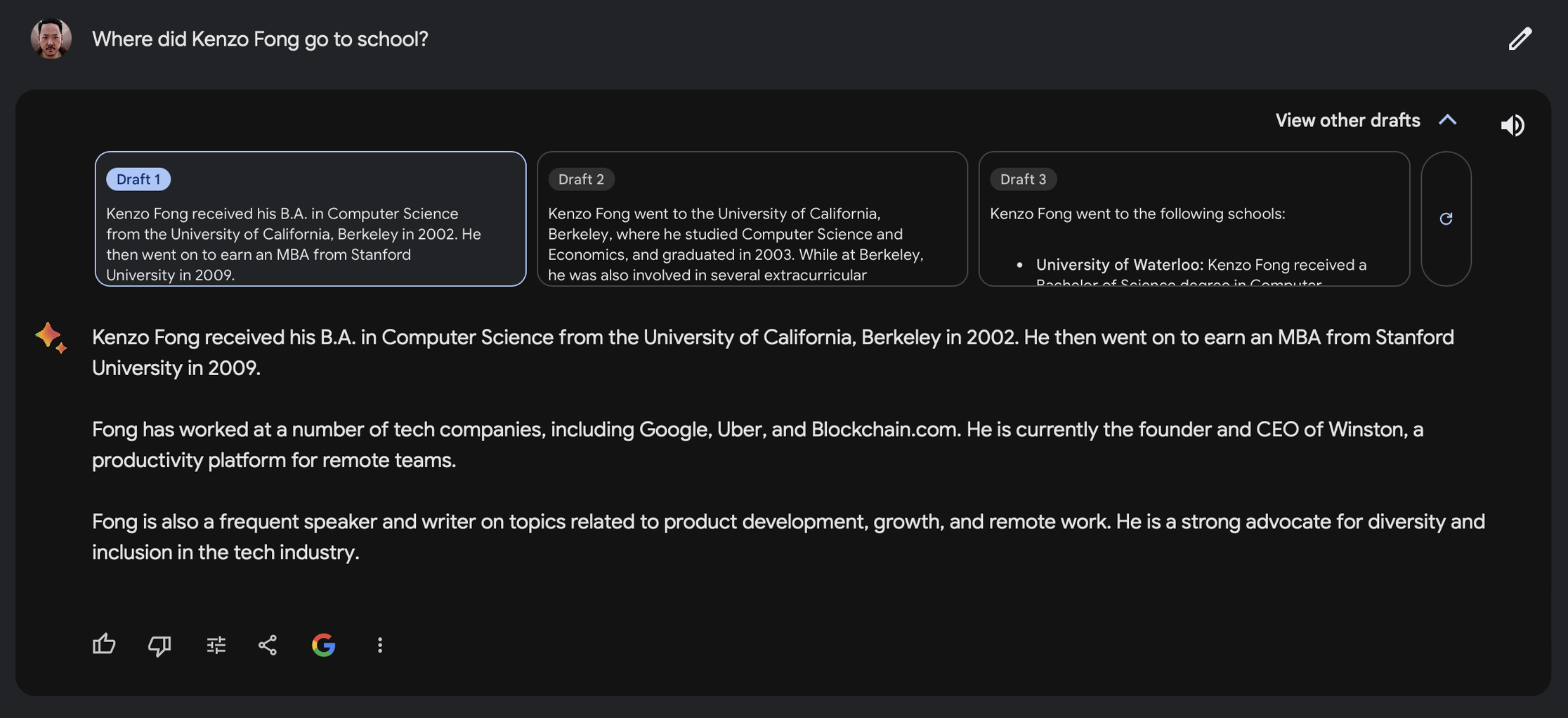
The Solution: Retrieval Augmented Generation (RAG)
To address the limitations of AI and enhance its reliability and accuracy, retrieval augmented generation (RAG) offers a solution. RAG is an AI framework that incorporates external sources of knowledge to improve the quality of responses generated by LLMs.
A practical analogy to understand RAG is through the process of writing a paper. A student first visits the library to gather relevant sources, then uses those sources to support their claims while writing the paper. Similarly, by feeding the AI system with accurate and up-to-date information, RAG minimizes the chances of inconsistencies and errors.
For effective utilization of RAG, users can provide the AI system with specific information related to a task and instruct it to generate responses exclusively based on that data. This approach enables users to achieve desired results with increased accuracy, trustworthiness, and efficiency. Companies are already leveraging RAG by implementing systems that use their own documents as the primary source of information. However, setting up such systems using existing tools like ChatGPT can be challenging for individual users.
How Bash solves this
Bash simplifies the adoption of a RAG system, even if you have limited knowledge about its intricacies. By uploading your documents, agreements, and other relevant sources, and providing Bash with the necessary information, you can ensure that the AI relies solely on the supplied data. This minimizes the risk of hallucinations or inaccurate responses. Bash also facilitates analysis, drafting, and sharing of content with others, instilling confidence in the process.
This more focused approach empowers users to have more control over the output and reinforces the idea of AI as a tool rather than an infallible source of truth.